
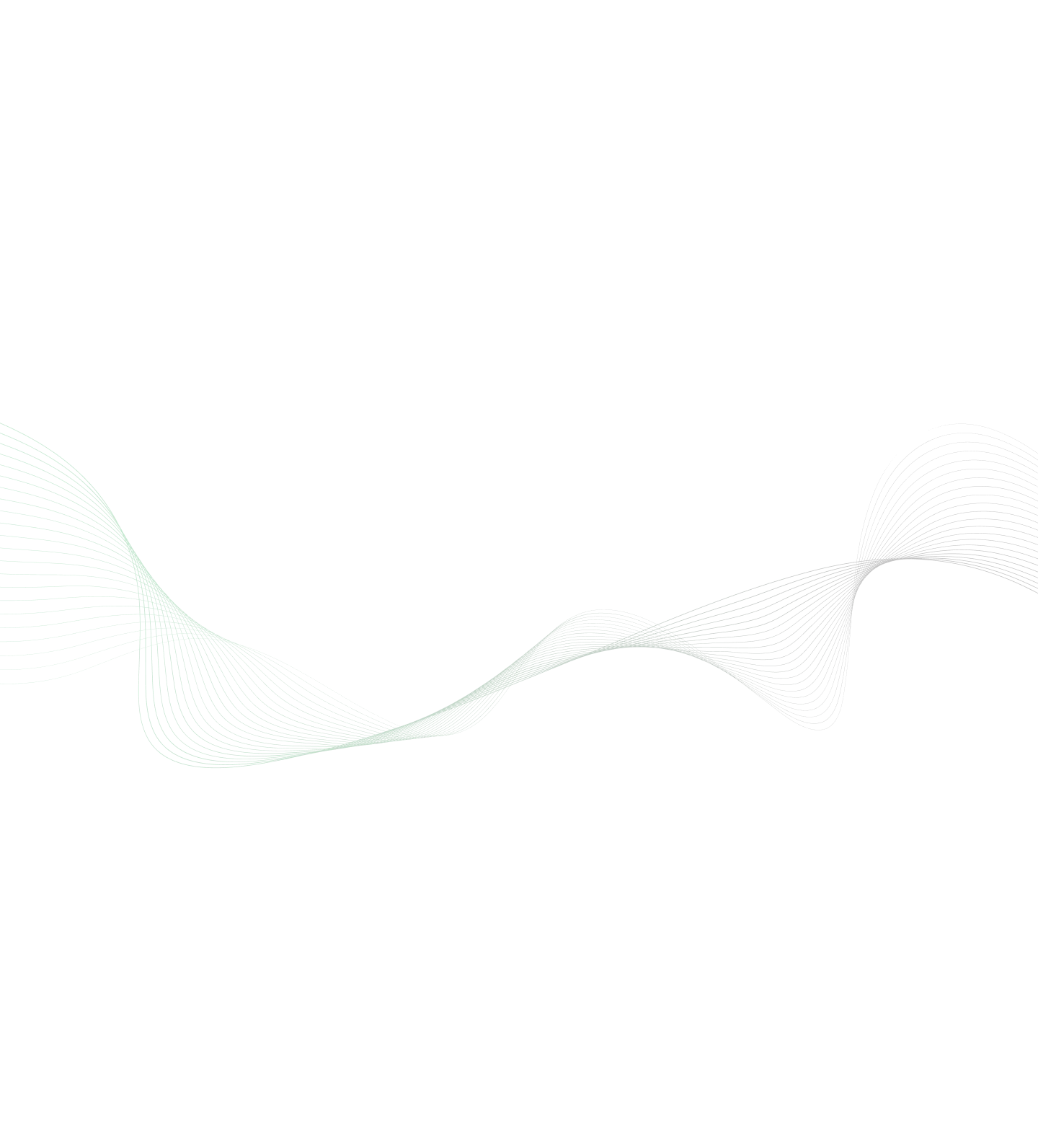
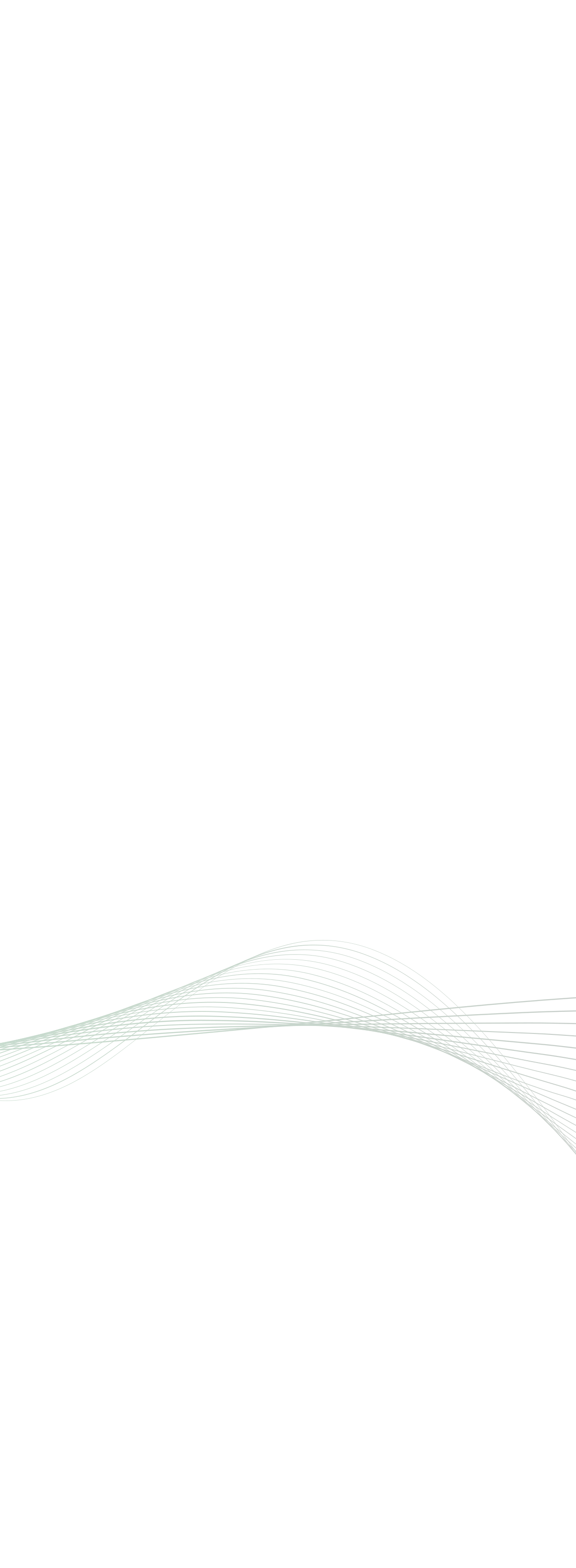
In the words of Gartner’s Vice President for Research, Fabrizio Biscotti- “Hyperautomation has shifted from an option to a condition of survival”. Technology continues to evolve and intelligent automation use-cases are emerging everywhere -- from enterprise workflows to software development processes in the “go-to-market” rush.[1]
Agile development, DevOps, and the constant progress in the underlying technology stack have wrought monumental changes in the way software products are engineered and deployed today. Software engineering teams especially SDETs now face the constant pressure to release fast and release often. Independent software vendors (ISV) are planning how to progress into a stage where hyperautomation impacts the entire product development process.
But what happens when we reach the speed limit cap of what traditional development practices allow? That’s when ISVs need to bring on-board Artificial Intelligence (AI) and Machine Learning (ML) technologies. This will facilitate the creation of autonomous processes and development paradigms that enable even greater acceleration. And on the path to create better digital products faster, infusing AI into the Quality Assurance (QA) practice may offer greater value.
New Quality Engineering Challenges AI Can Address in Product Development
As product development picks up at an unbelievable pace, emerging and novel quality engineering challenges need to be addressed. Some of the key challenges include:
- Predicting defect patterns to ensure more streamlined product development efforts resulting in lower project costs
- Enabling better forecasting of QA costs based on project sizes, better estimation of effort and transparent budgeting or billing in future projects
- Developing highly reusable test cases and test data to speed up time-to-market and reduce testing costs
- Acquiring and integrating feedback into the digital product development cycle to guarantee better customer satisfaction
QA will (obviously) play a critical role in ensuring that the digital products can meet customer expectations in every end use-case scenario. Intelligent testing, supported by AI, is an attractive way forward in this supercharged scenario.
Artificial Intelligence in Quality Engineering of Modern Digital Products
Let us examine how AI in testing can help ISVs address some core challenges in quality engineering for modern digital products:
AI-based Preventive Quality Assurance
Artificial intelligence and machine learning algorithms can learn from vast datasets of successful code. These algorithms can be used for similar functionality or user scenarios and quickly identify deviations in the coding exercise currently underway. They can evaluate the degree to which the deviation occurs and notify SDETs when the deviation is “likely” to create quality issues.
Software engineering teams can analyze data from historic data sets containing information about the root cause, the pattern of occurrence, impact or severity, etc. And AI systems can help minimize defects in future projects by providing data on possible defect scenarios beforehand.
AI in testing could predict which module or test scenario is likely to have more defects based on historic evidence from metrics such as Defect Removal Efficiency (DRE) and Defect Rejection Ratio (DRR). Focusing that bit more on these areas can help prevent defects.
Improve Quality, Estimate Better With AI-based Quality Engineering
Software engineering teams can leverage AI’s ability to scan and infer from a vast pool of data instantly. This will help development (and testing) efforts to be better budgeted. Artificial intelligence can be used to estimate the most accurate timelines for the completion of different stages of QA for a particular product development project.
Engineering VPs could get a better sense of the time and effort to deliver a product of desired quality. This can be taken after factoring in the development, testing, and rework effort. AI can provide these insights by analyzing vast swathes of historical data on similar projects. Thus, helping organizations to prepare transparent budgeting and billing processes.
Enhance Testing Speed and Granularity With Artificial Intelligence
Digital product quality assurance is no stranger to automation. Tons of test automation tools and platforms enable Quality Assurance professionals to easily automate test cases and speed up agility in software testing. But as products grow larger, their complexity increases.
Test strategies have to bake in considerations for integrations, interfaces, and modular architecture from approaches like Microservices. This complexity can stretch existing software test automation platforms to their limits. It’s also true that human insight still drives much of the output of test automation platforms and there’s a bandwidth constraint imposed due to that too.
However, with AI, this scenario could well be transformed. An algorithm-led AI can run 24X7 and for any time duration. It can identify gaps or issues with interfaces and integration points of large enterprise applications. Besides, it can help quality engineers with more comprehensive analysis with higher precision and accuracy.
The product engineering folks can prioritize where they expend their energy to fill the gaps identified. This could help them accelerate the certification and validation process and, ultimately, improve the time-to-market for these complex software products.
Intelligent and Self-Learning Test Scripts
Automated test scripts have made it easier for SDETs to test fast, expand test coverage, and relieve themselves of tedious tasks. Imagine the impact if test scripts for automation do not need to be specifically created or changed along the way. Imagine if they could evolve on their own through learning from similar scenarios. This is exactly what artificial intelligence promises for test scripts.
Through machine learning, the system understands how humans automate test cases depending on scenarios and the AI system can mimic that to build more efficient test scripts to launch faster.
Additionally, conversational tools like AI chatbots can constantly work with both customer stakeholders as well as development teams to understand and communicate feedback to the concerned teams. This could bring in more authenticity as feedback gets logged in the AI and doesn’t get missed. AI can enable greater test automation agility and impact.
Wayforward With Artificial Intelligence for Quality Engineering
Building a sustainable software product strategy necessarily means creating an efficient testing and quality assurance strategy. As modern digital products and applications hit the market ever-faster, ISVs will have no choice but to accelerate their own product development. Artificial intelligence can help them create powerful and scalable quality assurance practices and methodologies that ensure high-quality digital products get released at the speed of modern business.
Xoriant Artificial Intelligence for Quality Engineering
To meet the rising expectations of digital customers, your teams are embracing the agile approach, bringing software quality and testing into core engineering early on in the SDLC. Xoriant has a good handle on the new age of advanced quality engineering driven by digital transformation, DevOps, and cloudification along with Quality Engineering frameworks. We excel in Artificial Intelligence and data-driven testing, IoT edge testing, data migration, Blockchain, and RPA Testing.
Get specialized quality engineering for your success